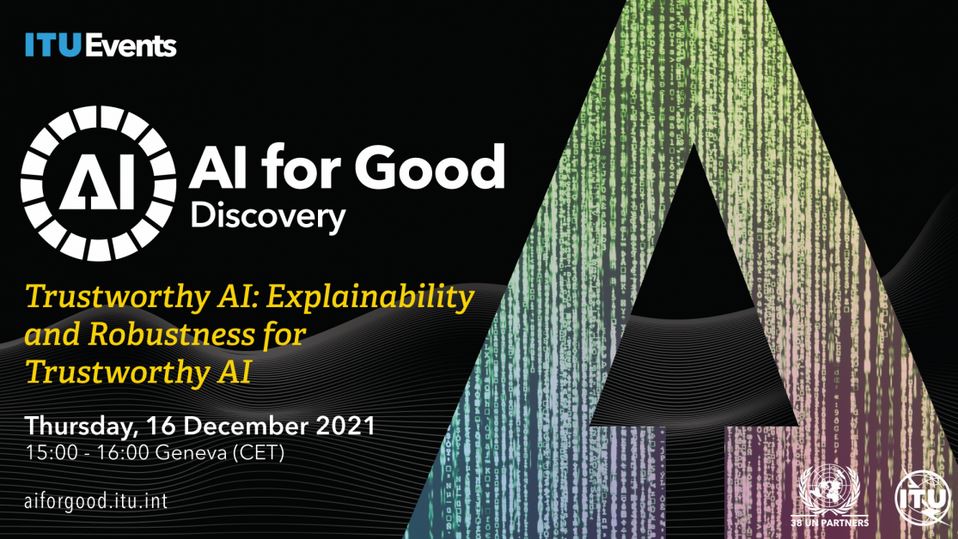
AI for Good Discovery. Trustworthy AI: Explainability and Robustness for Trustworthy AI, ITU Event, Geneva, CH [youtube]
Talk recorded live on 16.12.2021, 15:00 – 16:00 – see https://www.youtube.com/watch?v=NCajz8h13uU
Today, thanks to advances in statistical machine learning, AI is once again enormously popular. However, two features need to be further improved in the future a) robustness and b) explainability/interpretability/re-traceability, i.e. to explain why a certain result has been achieved. Disturbances in the input data can have a dramatic impact on the output and lead to completely different results. This is relevant in all critical areas where we suffer from poor data quality, i.e. where we do not have i.i.d. data. Therefore, the use of AI in real-world areas that impact human life (agriculture, climate, forestry, health, …) has led to an increased demand for trustworthy AI. In sensitive areas where re-traceability, transparency, and interpretability are required, explainable AI (XAI) is now even mandatory due to legal requirements. One approach to making AI more robust is to combine statistical learning with knowledge representations. For certain tasks, it may be beneficial to include a human in the loop. A human expert can sometimes – of course not always – bring experience and conceptual understanding to the AI pipeline. Such approaches are not only a solution from a legal perspective, but in many application areas, the “why” is often more important than a pure classification result. Consequently, both explainability and robustness can promote reliability and trust and ensure that humans remain in control, thus complementing human intelligence with artificial intelligence. Speaker: Andreas Holzinger, Head of Human-Centered AI Lab, Institute for Medical Informatics/Statistics Medizinische Universität Graz Moderators: Wojciech Samek, Head of Department of Artificial Intelligence, Fraunhofer Heinrich Hertz Institute
Watch the latest #AIforGood videos! https://www.youtube.com/c/AIforGood/v… Explore more #AIforGood content: AI for Good Top Hits https://www.youtube.com/playlist?list… AI for Good Webinars https://www.youtube.com/playlist?list… AI for Good Keynotes https://www.youtube.com/playlist?list… Stay updated and join our weekly AI for Good newsletter: http://eepurl.com/gI2kJ5 Discover what’s next on our programme! https://aiforgood.itu.int/programme/
Check out the latest AI for Good news: https://aiforgood.itu.int/newsroom/ Explore the AI for Good blog: https://aiforgood.itu.int/ai-for-good… Connect on our social media: Website: https://aiforgood.itu.int/ Twitter: https://twitter.com/ITU_AIForGood LinkedIn Page: https://www.linkedin.com/company/2651… LinkedIn Group: https://www.linkedin.com/groups/8567748 Instagram: https://www.instagram.com/aiforgood Facebook: https://www.facebook.com/AIforGood What is AI for Good? The AI for Good series is the leading action-oriented, global & inclusive United Nations platform on AI.
The Summit is organized all year, always online, in Geneva by the ITU with XPRIZE Foundation in partnership with over 35 sister United Nations agencies, Switzerland and ACM. The goal is to identify practical applications of AI and scale those solutions for global impact. Disclaimer: The views and opinions expressed are those of the panelists and do not reflect the official policy of the ITU.